Top Skills of High Performing HR Data Scientists
David Morgan of Micron Technology shares his overview of the HR Data Scientist role and a data scientist job description.
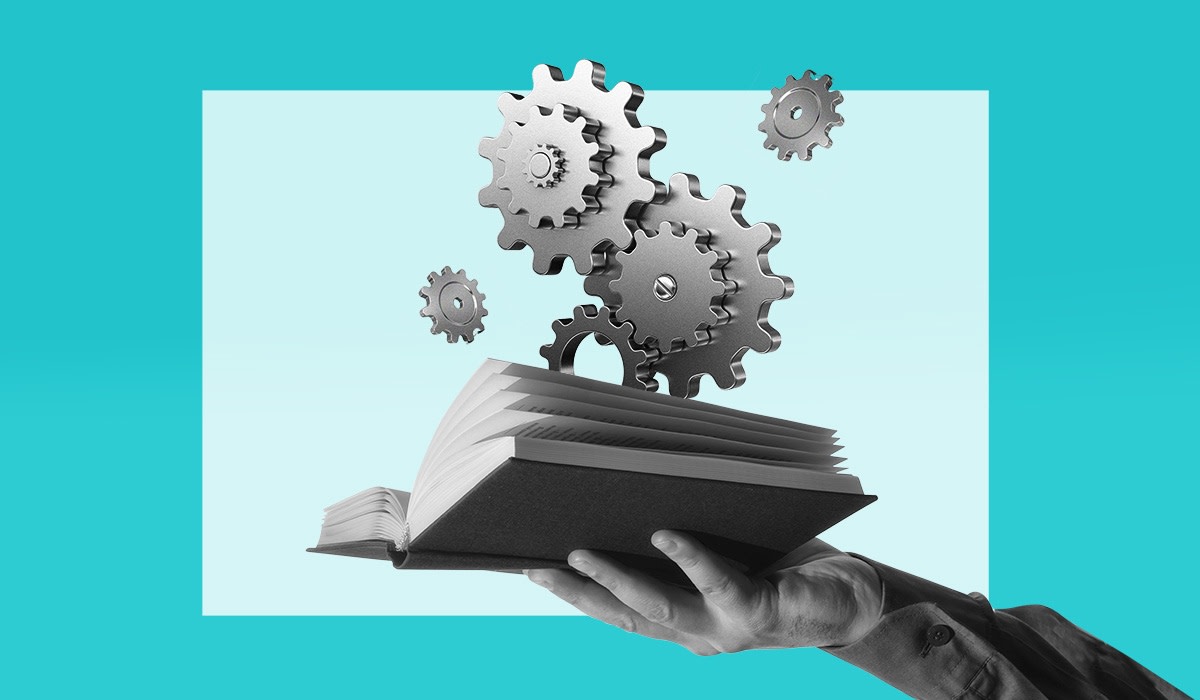
We are pleased to have David Morgan, Global Director of Workforce Analytics at Micron Technology, share his overview of the HR Data Scientist role below and a job description template that we encourage all to use in their hiring efforts.
The support of the CHRO and other key leaders can go a long way in ensuring organization-wide interest in people analytics, but you will need other enablers to evangelize adoption. Many roles contribute to successful people analytics enablement. In our research over the past year, we have found that–in addition to the analytics-focused CHRO, people analytics function leader, and HR business partner manager–the HR Data Scientist is also key to success.
The profile of a successful Human Resources (HR) Data Scientist is a unique one, both within HR, and across the field of data science more broadly.
Entry into the discipline requires at the very least strong data science skills, knowledge of research design principles, the ability to clearly explain technical concepts to people with non-technical backgrounds, and, of course, an interest in studying people in the workplace.
Mid- and senior-level HR Data Scientists must cover all these areas. Moreover, they are expected to serve as consultants and advisors by presenting clear, actionable recommendations to their stakeholders. Figure 1 shows a typical cycle consulting framework for an HR Data Scientist.
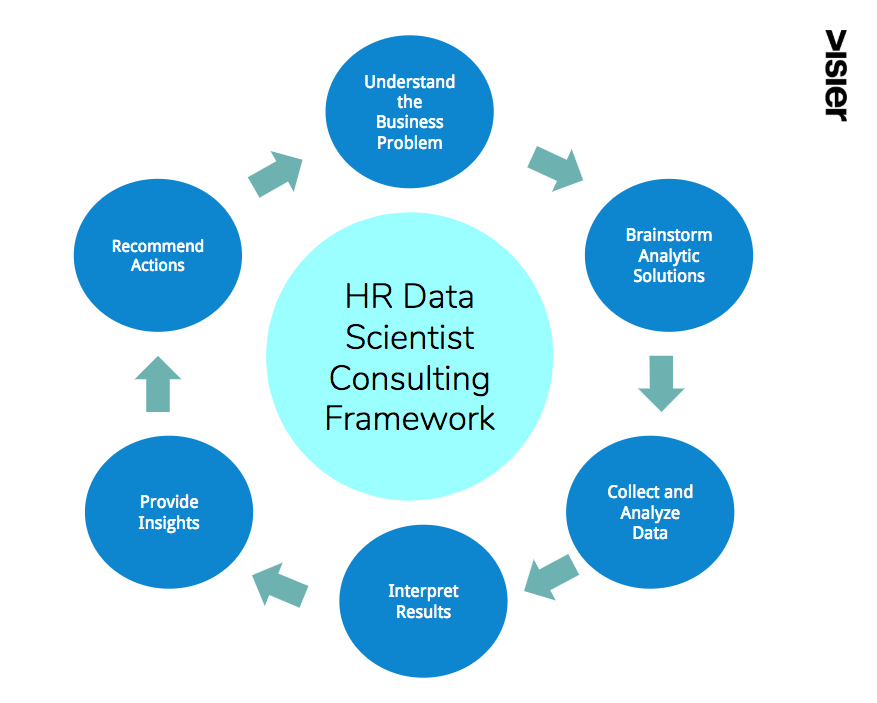
There are no one-size-fits-all experiential or educational backgrounds indicative of the most effective, proficient HR Data Scientists. To the contrary, HR data science teams (commonly referred to as Workforce Analytics, People Analytics, or People Science teams) are often multidisciplinary functions by design.
From policy researchers to particle physicists, there appears to be no limit to the types of fields and backgrounds welcomed into the world of HR data science, assuming one has the foundational knowledge and skills mentioned above. Cognitively diverse teams typically outperform cognitively homogenous ones—and HR data science leaders know it.
Role Overview
There tends to be significant overlap with requirements of data scientists in other functions, such as demonstrating robust data science skills and coding abilities. However, additional challenges related to analyzing people data in the context of HR are often encountered. For example, HR Data Scientists must:
Actively listen in order to understand (and ask questions about) people problems to be solved
Display strong non-technical communication skills such that business leaders are able to fully understand the meaning and implications of results
Have a firm understanding of labor and employment law considerations
Be adaptable in the face of frequent context-switching (e.g. coding vs. emailing vs. meetings, or recruiting vs. diversity vs. retention)
These are core competencies required for the HR-Data-Scientist-as-consultant model. When studying diversity in organizations, for example, the ubiquity of this problem (particularly in the high-tech industry) presents unique data challenges—which are often pervasive throughout the employee lifecycle—such as small sample size issues.
Diversity program evaluations, such as determining the effectiveness of diverse slate talent acquisition initiatives, can be especially difficult to confront in practice, because it can take several months or even years to collect enough data such that statistical inferences can be drawn. Considerations of practical significance (as opposed to statistical significance), external data sources, and qualitative feedback are extremely important when the business needs an answer in days, rather than months or years.
Active listening sessions with recruiters, qualitative feedback assessments of candidate experiences, and proactive investigations of available outside data sources may offer critical evidence for or against the effectiveness of a diverse slate initiative.
A high-performing HR Data Scientist will apply the competencies mentioned above, toward fulfilling these core expectations and responsibilities:
Collaborate with partners and stakeholders to understand people-related business problems, and gathering relevant people data to analyze
Utilize appropriate data science methodologies toward attracting, hiring, developing, and retaining qualified talent
Interpret statistical models that explain or predict outcomes related to hiring, productivity, engagement, and attrition
Prepare and deliver presentations with rich data visualizations and meaningful business insights and conclusions
Develop data science skills as new or improved techniques arise, and continue exploration of emerging methods and technologies in the analytics space
Demonstrating a healthy mix of soft skills and domain-specific knowledge, accompanied by a breadth of data science technical expertise, differentiates good HR data scientists from truly great ones.
The Difference Between the HR Data Scientist and I/O Psychologist
Notably, there is a large contingent of professionals from one social science discipline in particular—industrial/organizational (I/O) psychology—who are frequently drawn to HR data science.
As a branch of psychology centered on applying psychological principles to the workplace, I/O psychology practitioners focus on areas such as pre-employment testing and assessment, leadership development, talent acquisition, team effectiveness, total rewards, workplace safety, diversity, employee engagement, etc. The discipline as a whole has been ranked by U.S. News and World Report as a top science job in 2018.
While there is substantial overlap between HR data science and I/O psychology, the former tends to be heavily quantitative, whereas many aspects of the latter need not be. Both require subject matter expertise in the HR domain; however, an HR Data Scientist must exhibit quantitative skills, such as advanced statistical modeling, and technical skills related to data or software engineering on a near daily basis.
It may be somewhat confounding that a master’s degree or PhD is typically required to practice the discipline of I/O psychology. But this is not a requirement of an HR Data Scientist–a bachelor’s degree with a concentration in quantitative methods or relevant technical experience may be adequate for realizing full performance (which encompasses being able to fulfill the core responsibilities listed above).
HR Data Scientists Face Unique Data Issues and Projects
HR Data Scientists encounter a high frequency of data-related issues partially unique to the role. For example, the ability to apply so-called big data techniques to small data problems is a common necessity.
Even in large companies, HR datasets regularly pale in comparison to datasets from other areas and platforms (e.g., sales, manufacturing, etc.). Contemporary machine learning approaches may not work well (or may have to be adapted) in small sample scenarios; whereas, more traditional statistical or practical approaches may be better suited for these types of situations.
In addition, other areas may be more interested in prediction-heavy models. For example, predicting product defects prior to being shipped or forecasting a percent increase in sales in the next quarter. However, a vast number of HR data science problems are those which require elements of both prediction and inference.
Take, for example, one of the most common HR data science concepts of interest: regrettable turnover. It’s great to have the ability to predict if/when/which top performers are thinking about leaving your company, having the ability to infer why they might leave provides leaders with a fighting chance to intervene before it’s too late.
As someone who has studied regrettable turnover in different organizations, for different purposes, I have found that the core business problem or context can vary widely, and asking the right questions in order to understand the problem, is imperative. Are we concerned with high, or low, turnover? Do we care about identifying key drivers of attrition, or maximizing predictive power, or both? How will we intervene to prevent high-risk employees from leaving?
These are just a few examples of questions in which the answers will have important downstream implications for statistical modeling, interpretation, recommendations, and actions. These realities require careful thought around which methods or tools are best for a given HR-specific problem.
Hire the Right HR Data Scientist
The HR Data Scientist role is a fascinating study mainly because of the multi-dimensional nature of the job, requiring many different hats to be worn. The HR-Data-Scientist-as-consultant model (see Figure 1) serves as a useful framework for crafting specific roles and responsibilities appropriate for your organization. Please take advantage of this framework, as well as this sample job description that’s been provided as a template, to help you scale your organization’s own HR data science capabilities!
Get Outsmart content straight to your inbox
Subscribe to the People Insights Monthly newsletter for actionable insights and stories.
Subscribe now